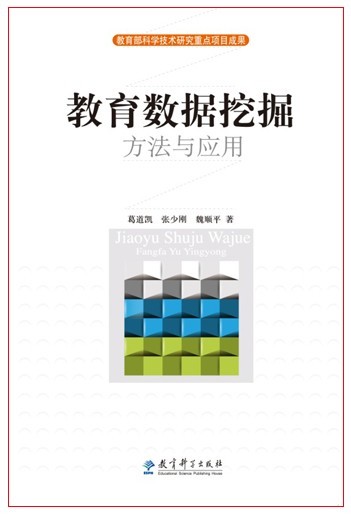
“Big data” was one of the technology buzzwords of 2012, used to describe the massive amount of data produced in the information explosion era and the technologies and innovations developed to deal with such data. Research by International Data Corporation (IDC) shows that the global volume of data was 0.49ZB in 2008, 0.8ZB in 2009, 1.2ZB in 2010, and grew to 1.82ZB in 2011. This is equivalent to over 200GB of data produced per human being. The arrival of the "big data" era was first mentioned by McKinsey, a global management consulting firm. McKinsey states: “Data have swept into every industry
and business function and are now an important factor of production….The use of big data will underpin new waves of productivity growth and consumer surplus.” The NMC Horizon Report - 2013 Higher Education Edition (February 2013) reports that learning analytics, an application of educational big data, is a major technology trend that will impact teaching, learning, and creative inquiry.
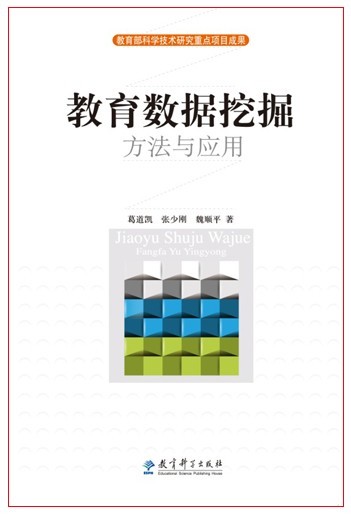
A new book entitled Educational Data Mining: Methods and Applications was published by Educational Science Publishing House at the end of 2012. It was co-authored by Professor Ge Daokai, director of the Department of Vocational and Adult Education at the Ministry of Education; Zhang Shaogang, vice president of the Open University of China (OUC); and Dr. Wei Shunping from the OUC Research Institute of Open and Distance Education.
The book focuses on “how to utilize the massive amount of educational data stored in educational software systems and convert it into information, knowledge and a basis for educational policy-making and teaching optimization to avoid ‘suffering from a thirst for information while drowning in an ocean of data’ . It predicts that data mining, which is extracting or “excavating” knowledge from massive data sets, will be conducive to bringing out the value of educational data and providing a basis for prudent policy-making. This book is the first domestic scholarly work that discusses the value of educational data mining in the era of big data.
The book explores two major aspects of educational data mining: methods and applications. It formulates a number of data mining models based on actual tasks and presents a host of empirical studies involving real-world problems in the research and practice of open and distance education that illustrate the great value of educational data mining.
Educational data mining is the process of converting the raw data from various educational systems into useful information. This information can be used by teachers, students, parents, educational researchers and developers of educational software. Teaching, management and research are the basic activities of educational institutions, and based on these three fields of application, educational data mining can be subdivided into e-learning data mining, e-management data mining and e-research data mining.
Educational data mining brings different benefits to different groups of people. For learners, its role is to suggest learning activities, resources and tasks that contribute to the improvement of their studies and good learning experiences. These suggestions can be obtained by analyzing their own learning behaviors and those of similar learners. For educators, its role is to provide more objective feedback so that they can optimize educational policy and processes, improve curriculum development, and organize teaching content and plans in line with learners’ circumstances.
Methods for educational data mining can be divided into five categories: statistical analysis and visualization, clustering (cluster and outlier analysis), projection (decision trees, regression analysis and timing analysis), relationship mining (association rule mining, sequential pattern mining and dependency mining), and text mining. Relationship mining and projection in particular have broad applications.
Through seven empirical studies on data mining in e-learning, e-management and e-research, the book gives readers a comprehensive understanding of the types of data available in the field of distance education, effective data mining methods and tools, and the knowledge patterns that can be obtained through mining. It draws the following basic conclusions:
First, proper use of data mining can help optimize education planning and management, improve the quality of education and teaching, and improve the design and development of educational software.
Second, as far as most educational institutions are concerned, the timely use of data mining in the educational process is not only possible but necessary.
Third, researchers can discover the current state and future direction of a given research field comprehensively, quickly and accurately by using data mining methods on various specialized databases.
Fourth, collecting and storing information related to educational processes, management processes and research processes is of great value.
The academic value of the book lies in two major aspects. The first is in expanding the application scope of data mining and innovating methods of system integration that optimize education policy. This provides new methods of analysis and measures for the reform and improvement of distance education. The second is in opening new domains of distance education research and helping scholars conduct more focused and effective research. This book will serve as a valuable and insightful reference for distance education administrators, teachers and researchers.
Big Data: A Revolution That Will Transform How We Live, Work, and Think (Viktor Mayer-Schonberger and Kenneth Cukier, January 2013) reveals three major trends in data processing in the era of big data: the completeness of data (as opposed to sampling), the ability to accept less than perfect accuracy, and the shift from causation to correlation. Readers will find these three trends reflected in the book Educational Data Mining: Methods and Applications.
By Wei Shunping from the OUC